Introduction
Persuasion Strategies in Advertisements
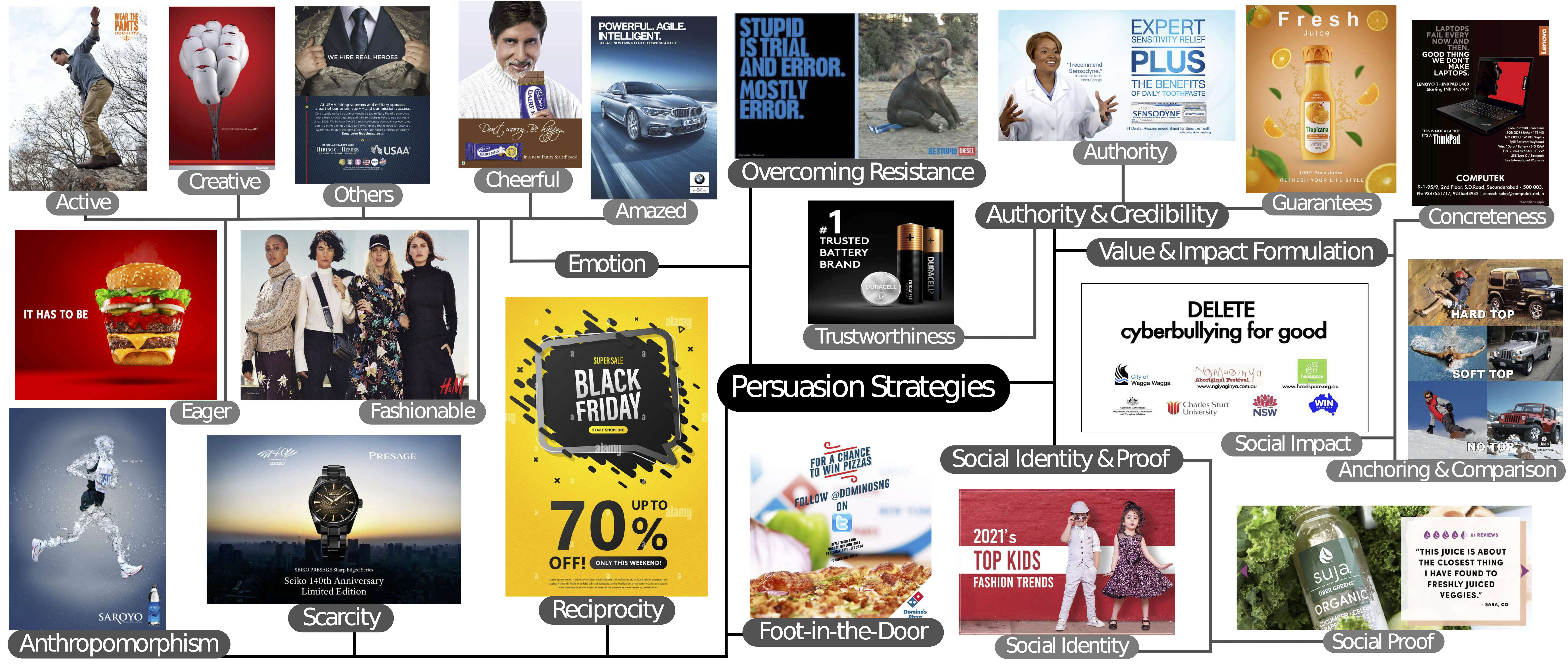
Modeling what makes an advertisement persuasive, i.e., eliciting the desired response from consumer, is critical to the study of propaganda, social psychology, and marketing. Despite its importance, computational modeling of persuasion in computer vision is still in its infancy, primarily due to the lack of benchmark datasets that can provide persuasion-strategy labels associated with ads. Motivated by persuasion literature in social psychology and marketing, we introduce an extensive vocabulary of persuasion strategies and build the first ad image corpus annotated with persuasion strategies. We then formulate the task of persuasion strategy prediction with multi-modal learning, where we design a multi-task attention fusion model that can leverage other ad-understanding tasks to predict persuasion strategies. Further, we conduct a real-world case study on 1600 advertising campaigns of 30 Fortune-500 companies where we use our model’s predictions to analyze which strategies work with different demographics (age and gender). The dataset also provides image segmentation masks, which labels persuasion strategies in the corresponding ad images on the test split. We publicly release our code and dataset.
Different Advertisements with Different Persuasive Strategies Selling Same Product
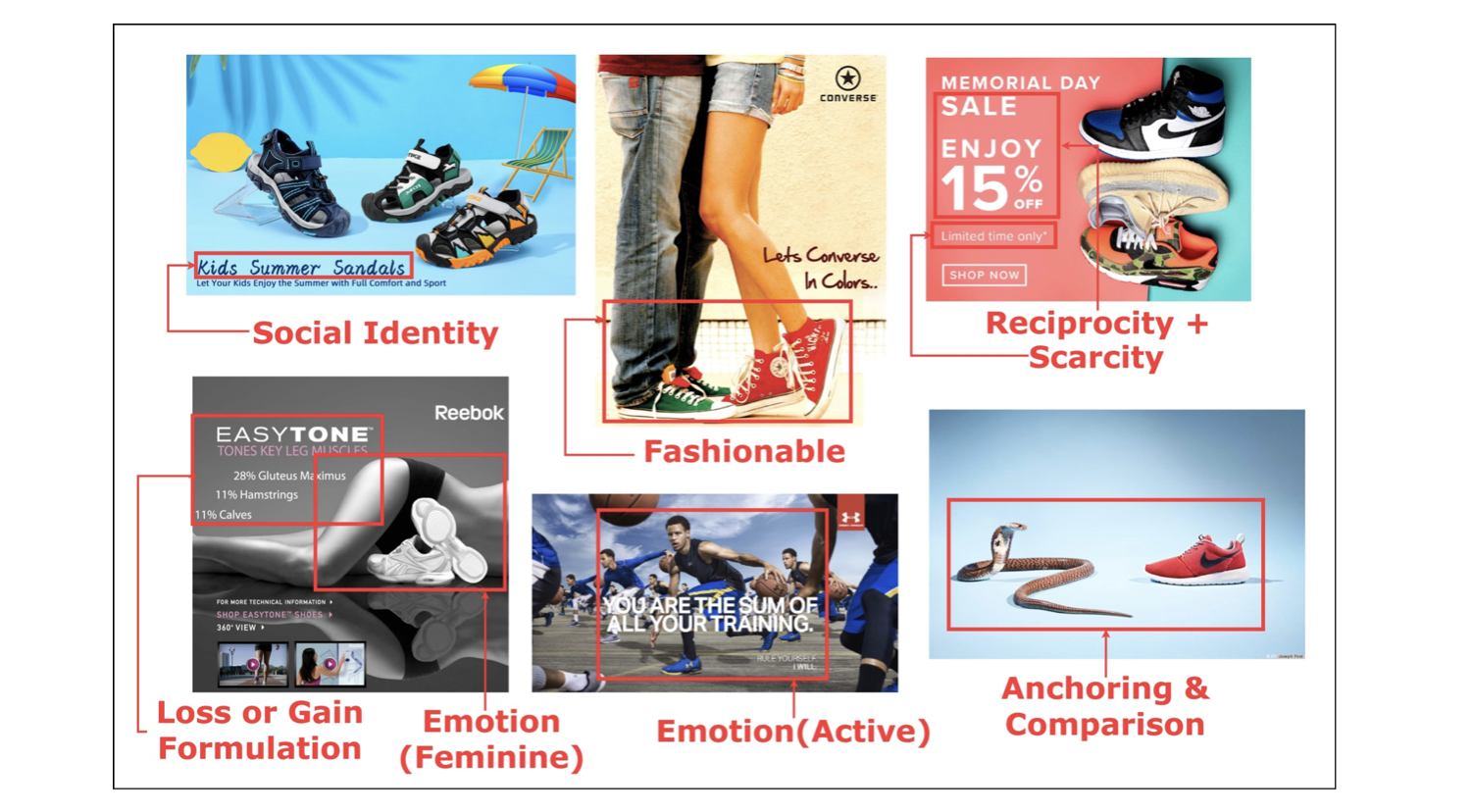
Explore Dataset
Distribution of Persuasion Strategies
Sample Segmented Images
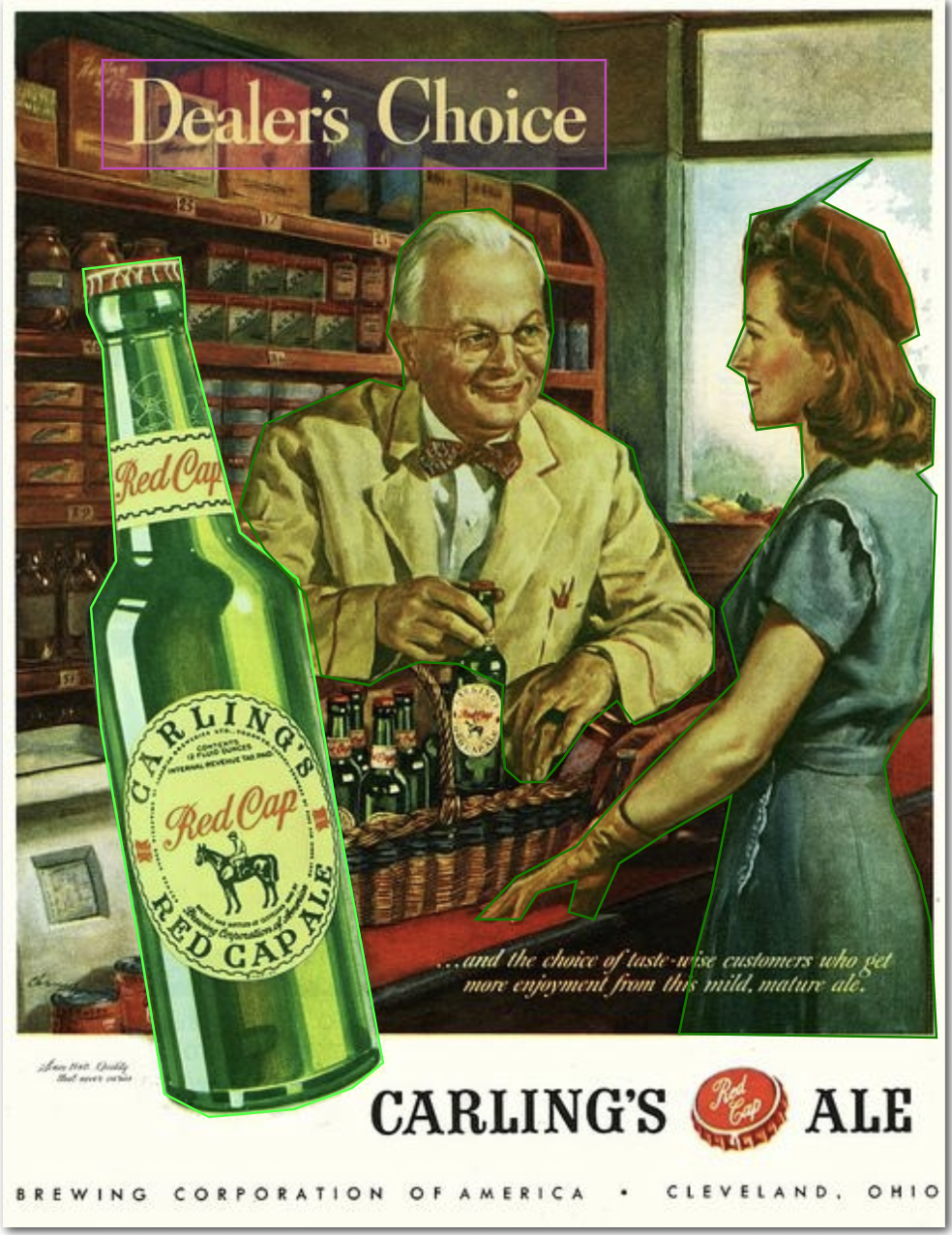
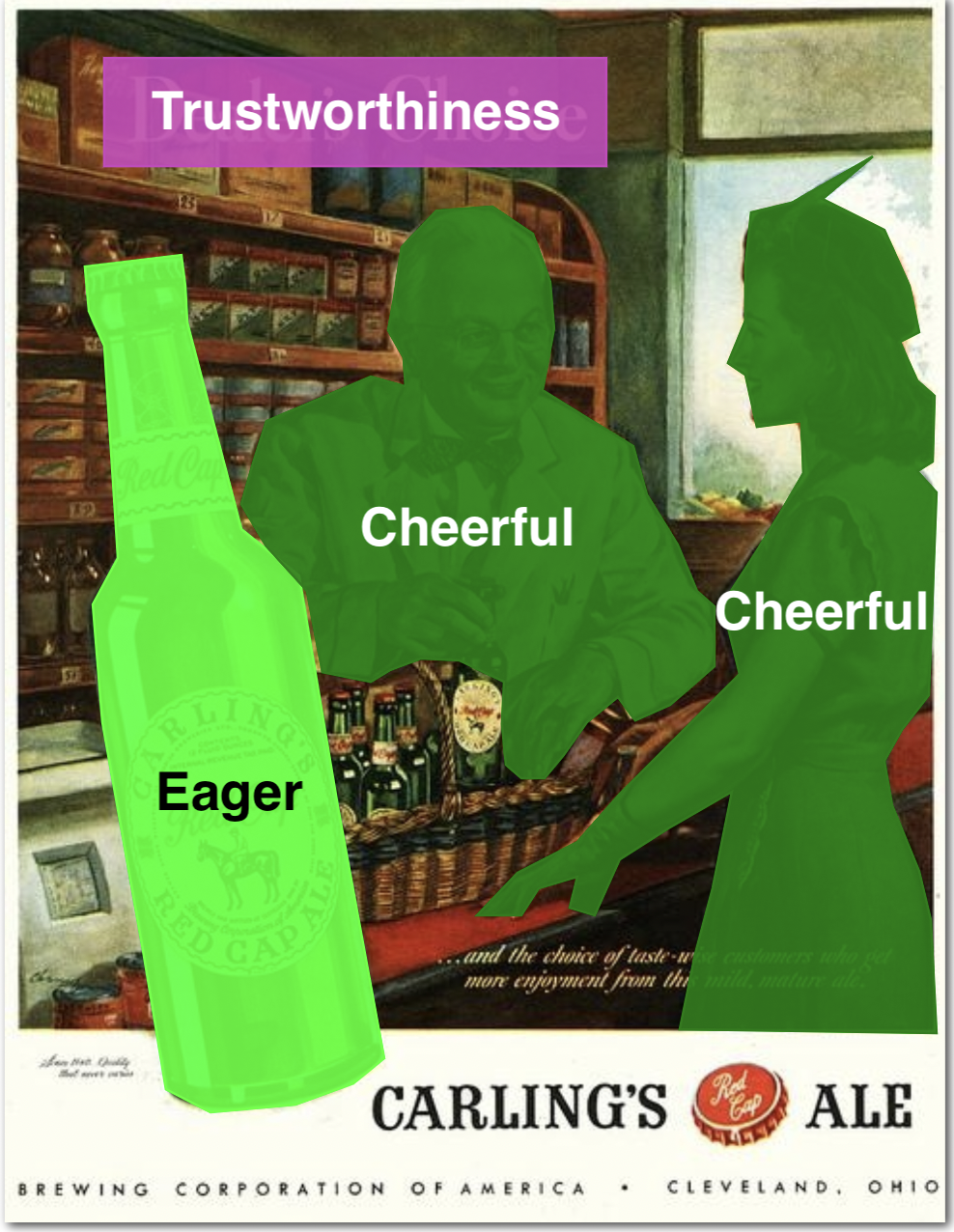
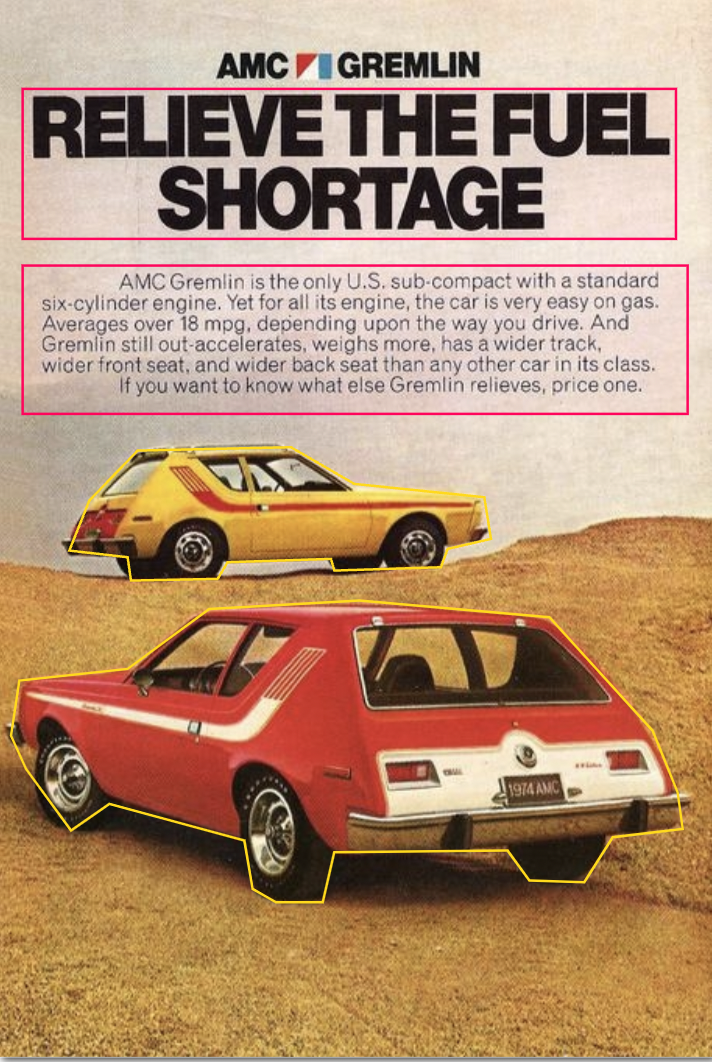
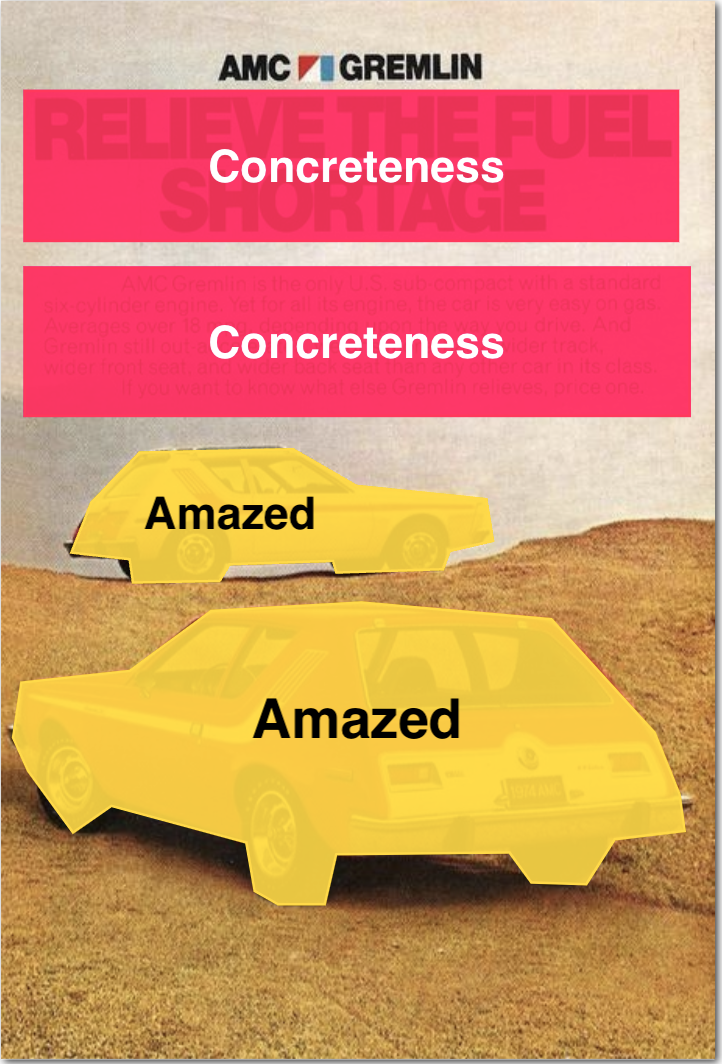
Pitt Ads Dataset
Pitt ads dataset contains an image dataset of 64,832 image ads, and a video dataset of 3,477 ads. This data contains annotations encompassing the topic and sentiment of the ads, questions and answers describing what actions the viewer is prompted to take and the reasoning that the ad presents to persuade the viewer. The Pitt Ads dataset can be donwloaded from here.
Get the Dataset
- Images
- Train Image Annotations
- Test Image Annotations
- Explore the Dataset with Category Wise Examples
- Segmentation Masks
- Model
- Read the Paper
Dataset Statistics
Persuasive Strategies | No. of Class Sample | Definition |
---|---|---|
Anchoring and comparison | 48 | A product’s value is strongly influenced by what it is compared to. |
Reciprocity | 186 | By obligating the recipient of an act to repayment in
the future, the rule for reciprocation begets a sense of future obligation, often unequal in nature |
Concreteness | 1007 | Using concrete facts, evidence to appeal to the logic of consumers |
Social Impact | 103 | Emphasizes the importance or bigger impact of a product |
Guarantees | 45 | Guarantees reduce risk and people try out such products more often. |
Trustworthiness | 157 | Trustworthiness indicated honesty and integrity of the source through tropes like years of experience, “trusted brand”, numbers and statistics |
Authority | 65 | Authority indicated through expertise, source of power, third-party approval, credentials, and awards |
Customer Reviews and Stories | 28 | Informational influence by accepting information obtained from others as evidence about reality, e.g., customer reviews and ratings |
Social Identity | 126 | Normative influence, which involves conformity with the positive expectations of “another”, who could be “another person, a group, or one’s self” (includes self-persuasion, fleeting attraction, alter-casting, and exclusivity) |
Scarcity | 64 | People assign more value to opportunities when they are less available. This happens due to psychological reactance of losing freedom of choice when things are less available or they use availability as a cognitive shortcut for gauging quality. |
Foot in the Door | 18 | Starting with small requests followed by larger requests to facilitate compliance while maintaining cognitive coherence. |
Reverse Psychology | 15 | Overcoming resistance (reactance) by postponing consequences to the future, by focusing resistance on realistic concerns, by forewarning that a message will be coming, by acknowledging resistance, by raising self-esteem and a sense of efficacy. |
Anthropomorphism | 37 | When a brand or product is seen as human-like, people will like it more and feel closer to it. |
Unclear | 148 | If the ad strategy is unclear or it is not in English |
Emotion | 238 | Aesthetics, feeling and other non-cognitively demanding features used for persuading consumers |
Amazed | 141 | Feeling surprised, astonished, awed, fascinated, intrigued |
Fashionable | 443 | Trendy, elegant, beautiful, attractive, sexy |
Feminine | 173 | Womanly, girlish |
Active | 256 | Feeling energetic, adventurous, vibrant, enthusiastic, playful |
Eager | 540 | Feeling of hunger, thirsty, passion |
Cheerful | 223 | Feeling delighted, happy, joyful, carefree, optimistic |
Creative | 402 | Inventive, productive |
Collaborators
1. Indraprastha Institute of Information Technology Delhi
2. Adobe Media & Data Science Research
3. Georgia Institute of Technology
4. The State University of New York, Buffalo
Contact
For any questions, issues, concerns, and comments, please email Yaman Kumar Singla at yamank@iiitd.ac.in
Terms of Use
If you use our data, please cite the following papers:
1. Singla, Yaman Kumar, Rajat Jha, Arunim Gupta, Milan Aggarwal, Aditya Garg, Ayush Bhardwaj, Tushar, Balaji Krishnamurthy, Rajiv Ratn Shah, and Changyou Chen. "Persuasion Strategies in Advertisements: Dataset, Modeling, and Baselines." arXiv preprint arXiv:2208.09626 (2022).
2. Hussain, Zaeem, Mingda Zhang, Xiaozhong Zhang, Keren Ye, Christopher Thomas, Zuha Agha, Nathan Ong, and Adriana Kovashka. "Automatic understanding of image and video advertisements."
In Proceedings of the IEEE conference on computer vision and pattern recognition, pp. 1705-1715. 2017.